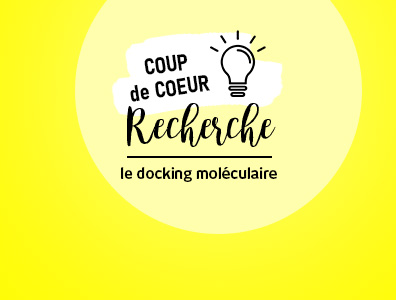
Coup de cœur RESEARCH
Spotlight on molecular docking
Molecular docking is a computational method used to predict the three-dimensional structure of molecular complexes, by simulating the interaction between a target molecule, often a protein, and a ligand, such as a potential drug. By identifying the most favorable position of the ligand in the active site of the target protein, i.e. the most stable position, molecular docking helps predict molecular interactions and improve their efficiency. In short, it reveals the optimal spatial arrangement of molecules when they bind to form a complex, as illustrated in Figure 1.
Figure 1: Principle of molecular docking, illustrating the interaction between a molecule (ligand) and a protein (Target) at its active site (Rectangle).
Since its development in the 1990s for the discovery of anti-HIV drugs1, molecular docking has become a standard and essential method in pharmaceutical research2 to predict protein-ligand interactions and aid drug design. Its applications extend into many other fields, where optimizing the spatial arrangement of macromolecules can improve their efficacy: in personalized medicine, to predict patient response to certain drugs according to their genetic and molecular profile; in the food industry, to optimize flavors and food additives; in agrochemistry, to design new, more specific pesticides and herbicides…
A few reminders about protein structure
Proteins, essential molecules as therapeutic targets, have active sites that enable specific interactions with other molecules. These active sites correspond to particular regions of the protein’s three-dimensional structure, where chemical reactions or molecular bonds take place. Their precise structure enables selective recognition and binding to target molecules, thus promoting biological functions. Interactions at the active site are key, stabilized by various forces such as hydrogen bonds and hydrophobic interactions, as illustrated in Figure 2.
Figure 2: representation of a protein interacting with a ligand (molecule shown in black) at its active site, illustrated in red and blue4.
To understand how proteins work, it’s important to understand their structure, organized into four hierarchical levels with active sites as essential elements. The primary structure, or sequence, corresponds to the linear succession of amino acids without reference to a spatial configuration. The secondary structure focuses on local motifs in the polypeptide chain, such as the α-helix and β-sheets, stabilized by hydrogen bonds between oxygen and hydrogen atoms. A visual representation of these structures is shown in Figure 3.
Figure 3: Schematic representation of the four hierarchical levels of protein structure5.
These secondary structural elements combine to form the protein’s three-dimensional structure, known as the tertiary structure, which is essential for its biological function and may include distinct functional motifs and domains. Moreover, proteins can combine into multimeric complexes, defining their quaternary structure, which involves the interaction of multiple protein subunits and plays a crucial role in many cellular processes. Understanding these fundamental concepts of protein structure, including active sites and their role in molecular interactions, is essential for the development of molecular docking. In fact, the method uses two approaches, based respectively on the study of the geometric and chemical complementarity of surfaces, and the calculation of the energy of the macromolecular complex.
Different types of docking depending on the type of molecular interaction
Protein-protein docking, shown in Figure 4, focuses on interactions between two distinct proteins. It is essential for understanding the formation of protein complexes involved in various cellular processes, such as transcription and translation regulation6,7. For example, in cancer research, this type of docking is used to study the interactions between proteins involved in regulating cell growth or tumor progression, offering prospects for the development of new targeted therapies8.
Figure 4: representation of docking between two proteins9.
Protein-peptide docking, shown in Figure 5, involves the interaction between a protein and a peptide. It is often used to study the regulatory mechanisms of cell signaling10,11. For example, it can be applied to the study of interactions between protein kinases and their substrate peptides, providing essential information for the design of drugs specifically targeting the signaling pathways involved in various diseases12.
Figure 5: illustration of the docking between a protein (molecule on the left) and a peptide (molecule on the right)13.
Finally, protein-ligand docking concerns the interaction between a protein and a small organic molecule called a ligand, also key to drug discovery3,14. It enables us to predict how small molecules interact with their protein targets, providing vital information for designing drugs that act in highly specific ways, reducing undesirable side effects15.
Rigid or flexible docking?
The choice between rigid or flexible approaches depends on the nature of the biomolecular system under study and the specific research objectives16. Rigid docking is preferred when proteins and ligands retain their initial conformations during complex formation, for stable interactions with few conformational changes17. When interactions involve significant conformational changes, flexible docking allows conformational movements to be taken into account to optimize intermolecular interactions and improve the accuracy of binding predictions18.
Figure 6 shows a schematic representation of these methods, in the case of docking between a protein and a ligand.
Figure 6: Rigid and flexible approaches19
Which software?
Computational tools are mobilized to predict and model interactions between different molecular entities, particularly protein-ligand complex interactions, to facilitate the drug design process by enabling researchers to predict the three-dimensional structures of complexes. Among the software, we can mention, Haddock 20Cluspro21 LightDock 22,InterEvDock323LZerD24 AutoDock Vina25and CABSDOCK26.
Artificial intelligence (AI) makes its contribution by improving docking performance. Methods based on machine learning, such as neural networks, are now widely used. For example, they can accurately predict ligand and protein conformations, and filter out potential binding candidates. These advances enhance the efficiency and accuracy of Docking simulations, opening up new perspectives in drug discovery. Software that incorporates these techniques includes AutoDock27, SWISS-MODEL28 and DeepDock29, which offer innovative solutions for molecular analysis and drug design.
Molecular docking at EBI
Within the EBInnov® research unit, two projects are exploiting these techniques to deepen our understanding of biological processes and open up new therapeutic perspectives.
Firstly, EBInnov® developed a biomimetic motif inspired by human fibronectin, a glycoprotein found in the extracellular matrix and playing a key role in cell adhesion. Using advanced bioinformatics techniques, notably the Alphafold algorithm30, the three-dimensional structure of this motif was determined. This structural resolution was then used to explore the interactions between the motif and extracellular matrix proteins, enabling a detailed understanding of their affinities and different possible conformations.
Then, the second subject involving docking, EBInnov® seeks to identify new therapeutic targets in the fight against melanoma. These cancers pose a major challenge because of their potential resistance to immunotherapy. The project aims to combine bioinformatics, experimental and molecular modeling approaches to understand resistance mechanisms and identify new therapeutic targets. Molecular docking makes it possible to explore the interactions between biomolecules involved in melanoma, paving the way for new therapeutic strategies.
And tomorrow?
The growing integration of artificial intelligence will further improve the efficiency of simulations, our understanding of biological processes and accelerate the development of new therapies.
Contacts :
Dr Jad EID, Professor of Bioinformatics and Biophysics at EBI
References :
(1) Agu, P. C.; Afiukwa, C. A.; Orji, O. U.; Ezeh, E. M.; Ofoke, I. H.; Ogbu, C. O.; Ugwuja, E. I.; Aja, P. M. Molecular Docking as a Tool for the Discovery of Molecular Targets of Nutraceuticals in Diseases Management. Sci Rep 2023, 13 (1), 13398. https://doi.org/10.1038/s41598-023-40160-2.
(2) Meng, X.-Y.; Zhang, H.-X.; Mezei, M.; Cui, M. Molecular Docking: A Powerful Approach for Structure-Based Drug Discovery. Curr Comput Aided Drug Des 2011, 7 (2), 146-157.
(3) Torres, P. H. M.; Sodero, A. C. R.; Jofily, P.; Silva-Jr, F. P. Key Topics in Molecular Docking for Drug Design. Int J Mol Sci 2019, 20 (18), 4574. https://doi.org/10.3390/ijms20184574.
(4) Liao, C.; Peach, M. L.; Yao, R.; Nicklaus, M. C. Molecular Docking and Structure-Based Virtual Screening. In In Silico Drug Discovery and Design; Future Science Book Series; Future Science Ltd, 2013; pp 6-20. https://doi.org/10.4155/ebo.13.181.
(5) Simon, M. Protein structures. Pharmacy course. https://www.cours-pharmacie.com/biochimie/structures-des-proteines.html (accessed 2024-02-08).
(6) Grosdidier, A. Design of docking software and applications in the search for new active molecules.
(7) Kurcinski, M.; Kmiecik, S.; Zalewski, M.; Kolinski, A. Protein-Protein Docking with Large-Scale Backbone Flexibility Using Coarse-Grained Monte-Carlo Simulations. Int J Mol Sci 2021, 22 (14), 7341. https://doi.org/10.3390/ijms22147341.
(8) Férey, N.; Bouyer, G.; Martin, C.; Drif, A.; Bourdot, P.; Ammi, M.; Nelson, J.; Burkhardt, J.-M.; Autin, L. Protein Docking In Virtual Reality. Une Approche Hybride et Multimodale. Revue des Sciences et Technologies de l’Information – Série TSI : Technique et Science Informatiques 2009, 28 (8), 983-1015. https://doi.org/10.3166/tsi.28.983-1015.
(9) BioCIS – Biomolecules: Design, Isolation and Synthesis – Protein-Ligand Interactions. https://www.biocis.universite-paris-saclay.fr/?Interaction-Prot-Prot-900 (accessed 2024-02-08).
(10) Kurcinski, M.; Badaczewska–Dawid, A.; Kolinski, M.; Kolinski, A.; Kmiecik, S. Flexible Docking of Peptides to Proteins Using CABS–dock. Protein Sci 2020, 29 (1), 211-222. https://doi.org/10.1002/pro.3771.
(11) Chevrollier, N. Development and application of a fragment docking approach to model interactions between proteins and single-stranded RNA.
(12) Kaidanovich-Beilin, O.; Eldar-Finkelman, H. Peptides Targeting Protein Kinases: Strategies and Implications. Physiology 2006, 21 (6), 411-418. https://doi.org/10.1152/physiol.00022.2006.
(13) Protein-Peptide Docking – Profacgen. https://www.profacgen.com/protein-peptide-docking.htm (accessed 2024-02-08).
(14) Hernández-Santoyo, A.; Tenorio-Barajas, A. Y.; Altuzar, V.; Vivanco-Cid, H.; Mendoza-Barrera, C.; Hernández-Santoyo, A.; Tenorio-Barajas, A. Y.; Altuzar, V.; Vivanco-Cid, H.; Mendoza-Barrera, C. Protein-Protein and Protein-Ligand Docking. In Protein Engineering – Technology and Application; IntechOpen, 2013. https://doi.org/10.5772/56376.
(15) Grosdidier, A. Design of a docking software and applications in the search for new active molecules.
(16) Agrawal, P.; Singh, H.; Srivastava, H. K.; Singh, S.; Kishore, G.; Raghava, G. P. S. Benchmarking of Different Molecular Docking Methods for Protein-Peptide Docking. BMC Bioinformatics 2019, 19 (13), 426. https://doi.org/10.1186/s12859-018-2449-y.
(17) Ketata, M. A.; Laue, C.; Mammadov, R.; Stark, H.; Wu, M.; Corso, G.; Marquet, C.; Barzilay, R.; Jaakkola, T. S. DiffDock-PP: Rigid Protein-Protein Docking with Diffusion Models; 2023.
(18) de Oliveira, E. B. Molecular simulations applied to lipase-catalyzed flavonoid acetylation: influence of lipase and flavonoid structures and on bioconversion regioselectivity.
(19) Mohanty, M.; Mohanty, P. S. Molecular Docking in Organic, Inorganic, and Hybrid Systems: A Tutorial Review. Monatsh Chem 2023, 154 (7), 683-707. https://doi.org/10.1007/s00706-023-03076-1.
(20) van Zundert, G. C. P.; Rodrigues, J. P. G. L. M.; Trellet, M.; Schmitz, C.; Kastritis, P. L.; Karaca, E.; Melquiond, A. S. J.; van Dijk, M.; de Vries, S. J.; Bonvin, A. M. J. J. The HADDOCK2.2 Web Server: User-Friendly Integrative Modeling of Biomolecular Complexes. Journal of Molecular Biology 2016, 428 (4), 720-725. https://doi.org/10.1016/j.jmb.2015.09.014.
(21) Desta, I. T.; Porter, K. A.; Xia, B.; Kozakov, D.; Vajda, S. Performance and Its Limits in Rigid Body Protein-Protein Docking. Structure 2020, 28 (9), 1071-1081.e3. https://doi.org/10.1016/j.str.2020.06.006.
(22) Jiménez-García, B.; Roel-Touris, J.; Barradas-Bautista, D. The LightDock Server: Artificial Intelligence-Powered Modeling of Macromolecular Interactions. Nucleic Acids Res 2023, 51 (W1), W298-W304. https://doi.org/10.1093/nar/gkad327.
(23) Quignot, C.; Postic, G.; Bret, H.; Rey, J.; Granger, P.; Murail, S.; Chacon, P.; Andreani, J.; Tuffery, P.; Guerois, R. InterEvDock3: A Combined Template-Based and Free Docking Server with Increased Performance through Explicit Modeling of Complex Homologs and Integration of Covariation-Based Contact Maps. Nucleic Acids Research 2021, 49. https://doi.org/10.1093/nar/gkab358.
(24) Christoffer, C.; Bharadwaj, V.; Luu, R.; Kihara, D. LZerD Protein-Protein Docking Webserver Enhanced With de Novo Structure Prediction. Frontiers in Molecular Biosciences 2021, 8.
(25) Martz, F. Development of a new docking method based on enzymatic mechanisms and guided by prosthetic groups.
(26) Kurcinski, M.; Pawel Ciemny, M.; Oleniecki, T.; Kuriata, A.; Badaczewska-Dawid, A. E.; Kolinski, A.; Kmiecik, S. CABS-Dock Standalone: A Toolbox for Flexible Protein-Peptide Docking. Bioinformatics 2019, 35 (20), 4170-4172. https://doi.org/10.1093/bioinformatics/btz185.
(27) Grosdidier, A. Design of a docking software and applications in the search for new active molecules.
(28) Mezhoud, K. Docking Principles and methods.
(29) Gentile, F.; Agrawal, V.; Hsing, M.; Ton, A.-T.; Ban, F.; Norinder, U.; Gleave, M. E.; Cherkasov, A. Deep Docking: A Deep Learning Platform for Augmentation of Structure Based Drug Discovery. ACS Cent. Sci. 2020, 6 (6), 939-949. https://doi.org/10.1021/acscentsci.0c00229.
(30) Jumper, J.; Evans, R.; Pritzel, A.; Green, T.; Figurnov, M.; Ronneberger, O.; Tunyasuvunakool, K.; Bates, R.; Žídek, A.; Potapenko, A.; Bridgland, A.; Meyer, C.; Kohl, S. A. A.; Ballard, A. J.; Cowie, A.; Romera-Paredes, B.; Nikolov, S.; Jain, R.; Adler, J.Back, T.; Petersen, S.; Reiman, D.; Clancy, E.; Zielinski, M.; Steinegger, M.; Pacholska, M.; Berghammer, T.; Bodenstein, S.; Silver, D.; Vinyals, O.; Senior, A. W.; Kavukcuoglu, K.; Kohli, P.; Hassabis, D. Highly Accurate Protein Structure Prediction with AlphaFold. Nature 2021, 596 (7873), 583-589. https://doi.org/10.1038/s41586-021-03819-2.